Marketers always want to know whether the time, money, and effort they spend on marketing activities is generating conversions from users. To find out, you need to be able to differentiate organic traffic from the impact of paid advertising campaigns.
In mobile app advertising attribution, the last-touch point model is considered a popular model that most companies use to evaluate the effectiveness of their campaigns. However, this model still has limitations when it always emphasizes the role of the main touch point, while each touch point has certain contributions to creating conversions.
According to the basic marketing funnel, users often tend to search for the product they are interested in on Google channel first to learn about its features, uses or financial suitability, obviously Google has given them almost all the information to love that product. Then the ads about that product on Facebook gradually appear and have discount offers that make users convert immediately. Thus, considering Facebook as a channel that completely brings conversion seems unfair to Google, requiring a model that brings more insights into the actual effectiveness of each channel.
The challenge here is that using traditional attribution alone is not enough to infer causation, or rather, is inaccurate attribution. Furthermore, there may be additional issues related to marketing budgets and planning from incomplete marketing performance measurement.
In an era where traditional measurement methods are becoming less effective due to changes in data privacy, the technology landscape is evolving, and there is a growing need for solutions that accurately measure and optimize app marketing campaigns. Fortunately, incrementality can help you address these challenges.
What is the Incrementality Model in Marketing?
Lift can measure the impact of an ad on conversions compared to organic performance without the ad. It is extremely reliable because it effectively eliminates the impact of organic traffic. The essence of lift is creating two statistically similar groups. One group is exposed to ads, while the other is not. By comparing the difference in conversions between these groups, you can measure lift.
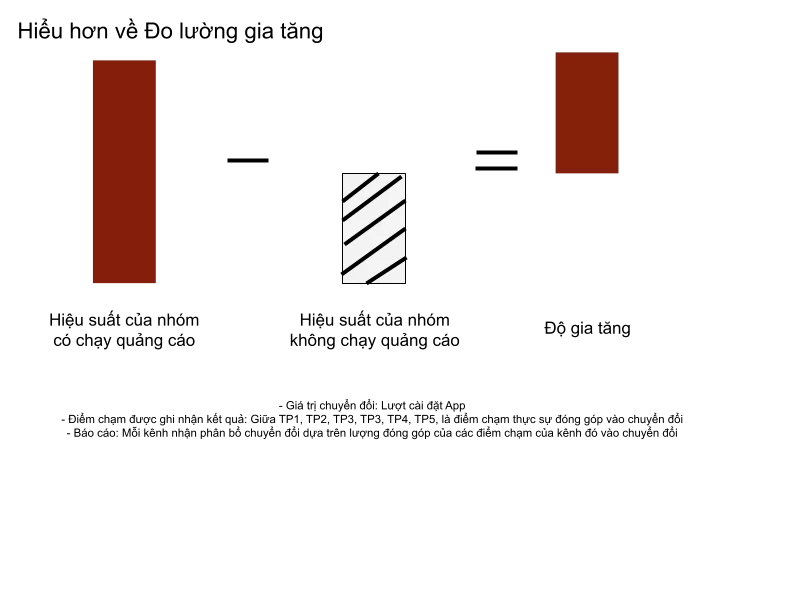
The concept of incremental measurement is further elaborated into two methods: the augmented method and the probability score method (PSM). The augmented method is based on the control of random variables, in which we conduct experiments with two randomly generated groups, only one of which is exposed to the advertisement. Although the augmented method provides accurate information about the advertising performance, it is not cost-effective and time-consuming because it has to be implemented in the field.
To address these issues, the probability score method takes a different approach. The calculation of the boost using this method requires sufficient data from previous executions. From this data, we can calculate the probability score and obtain two statistically similar groups. The difference in the number of conversions between these two groups is used as an index of the boost.
How to measure incrementality
There are many ways to measure growth, but experimental and observational methods are the two main methods. An experimental study divides users into a test group and a control group to compare the performance of the two groups and measure growth, while an observational study uses historical data to make causal inferences.
Experimental methods produce more accurate results, but they are also difficult. For example, you need data science experts on your team to create two groups with similar characteristics. However, no matter how carefully planned, it is nearly impossible to make two groups exactly the same. To handle such situations, you can take help from Meta and Google's Randomized Controlled Trials (RCTs) for incremental testing, which randomly assigns users to two groups.
However, experiments are not without their drawbacks – time, cost, and resources. Turning off ads for the control group during the study means missing out on sales opportunities. Furthermore, it takes time to validate and analyze the results, meaning that applying yesterday’s data to today’s decisions is not an option. This is why many martech tools like Airbridge have chosen to work with observational methods, which provide machine learning-based insights into user behavior.
1. Experimental: Meta & Google
Leading advertising platforms Meta and Google offer lift measurement tools that control how ads are shown to groups with similar characteristics. Based on experiments and real-world data, not only are the results standardized, accurate, and reliable, but they also effectively demonstrate causal effects. Let’s take a deeper look at how each advertising platform measures lift.
Meta’s Profitability Tests help you see the true value of your Facebook ads. This experiment splits your chosen target audience into groups that have and have not seen your Facebook ads to understand their causal impact on specific business goals like brand awareness or sales. The control group here would be people who match your target audience but are kept away from your ads.
There are 3 types of profit checks you can run on Meta:
- Conversion Lift tests: See how much additional lift your Facebook ads get for conversion goals.
- Brand Lift tests: Explore the additional impact of Facebook ads on brand awareness.
- Experiments: Answer predefined questions about your Facebook ads.
On Google, you can do two types of profitability checks:
- Check Brand Lift: Measure the effectiveness of your video ads.
- Conversion Lift: Measure the number of conversions, website visits, and other actions directly caused by your audience seeing your ads.
2. Observational: Combining & Adjusting Probability Scores
- Matching
To make a causal inference using observational data, the matching method is used to randomize the user groups and thereby minimize selection bias. With the matching method, the treatment and control groups can be adjusted to maintain a balanced distribution of variables.
For example, Heidi could match users in the treatment group with users in the control group based on similarity in observable characteristics, such as hours spent on smartphones per day. By removing all unmatchable users from the analysis, the distribution of this variable could be balanced. Can we consider these two groups similar? There are many other variables such as gender, age, annual income, and more. Many variables need to be considered in order to combine to create two similar groups.
Users can be matched differently depending on the variables chosen for comparison. When considering similarity in hours spent on smartphones, Sunny would be matched with Alex. But when considering similarity in annual income, she would be matched with Dana. How to match users considering multiple variables at once? Euclidean distance may be the answer. Euclidean distance is a formula for determining the similarity between rows of data with numerical values. But the problem with Euclidean distance is that it does not consider the difference in scale across variables, and therefore variables with large values will dominate the distance measure.
Based on the Euclidean distance, Sunny and Dana will be matched. The difference in hours spent on smartphones between Sunny and Dana is 12 hours, and between Sunny and Alex, the difference is 1 hour. The difference in annual income is $1,000 between Sunny and Alex and $2,000 between Sunny and Dana. The annual income variable has a larger numeric value than the hours spent on smartphones variable, resulting in the latter variable dominating the distance measure.
That is why Sunny is matched with Dana, not Alex, according to the Euclidean distance. This result is contrary to logic. Logically, the number of hours spent on smartphones should be the deciding variable in determining similarity between users. All three users belong to a similar income group, while the difference in hours spent on smartphones shows a big difference between the lifestyles of the users. This is why Heidi needs the help of another statistical method for matching.
- Propensity Score Matching (PSM)
Probability score matching (PSM) is another popular technique that uses scores to estimate the probability that a user is exposed to a particular ad. After matching users with similar probability score values from the test and control groups, all unmatched users are removed and the true impact of an ad is found by comparing the performance of the two groups.
For example, a probability score of 0.3 indicates that the probability of a user being exposed to an ad is 0.3 (30%), regardless of whether the user was actually exposed to the ad.
For another example, a user from the group exposed to the ad (treatment group) with a probability score of 0.15 is matched with a user from the group not exposed to the ad (control group) with the most similar probability value, in this case the user with a probability score of 0.1. The users who are not matched are removed from the analysis. Thus, the two groups become statistically similar. Everything is now ready to learn about the real effectiveness of the ad. Heidi can calculate the difference in sales between the two groups that occurred during the selected period. The difference is the real effect that the ad had on sales.
How can the Incrementality model help you?
- Validate your marketing budget
Profit is the ultimate business goal. To achieve that, marketers need to ensure that the return on investment is high and that such results are driven by marketing activities. The problem is that the last-touch attribution (LTA) model, which is now considered the industry standard, has limitations in attributing causal effects because it assigns 100% of credit to a single last touch for a conversion. This model oversimplifies the complex user journey and ignores the contribution of multiple prior touchpoints as well as the user’s underlying propensity to convert.
Fortunately, measuring incrementality can be a way to overcome the limitations of LTA, highlighting the importance of marketing campaigns in generating conversions. If you can demonstrate that conversions wouldn’t have happened without your marketing efforts, you can justify why your company needs to invest in marketing.
- Optimize marketing mix campaign
The increase also helps in channel-level analysis. By identifying higher performing channels, you can focus on advertising platforms that deliver tangible results, thereby preventing wasted budget and improving marketing performance.
There is always overlap between organic traffic and paid conversions, but the dividing line is often blurred, leading to less informed decisions. This is where incrementality measurement comes in, revealing whether your marketing mix is creating real value. Specifically, it provides answers to the following questions:
- What happens if you stop running ads on a particular channel?
- What role did a specific channel, campaign, or content play in increasing revenue?
- Which channels, platforms, publishers, or campaigns have the biggest impact on metrics like profit and lifetime customer value (LTV)?
- Which channels should you spend more or less on to maximize performance?
- Working with incrementality allows you to get the most accurate, actionable insight into the impact of your marketing efforts. Not only can you see the correlation between touchpoints and conversions, you can also best identify the best paid ad sets across different channels.
Why measure growth?
The goal of every marketer is to get the most accurate and comprehensive view of marketing performance and drive growth. While the LTA model has been widely requested for its convenience, it is not a perfect way to measure marketing performance, nor is it the only way, especially when it comes to assessing the true strength of your marketing efforts. Enhanced measurement provides a comprehensive view to identify the most effective campaigns and optimize paid advertising strategies.